Vasilis Katos, Bournemouth University
It’s a crime story fit for the digital era. It was recently reported that a number of restaurants in New York had been targeted by internet scammers threatening to leave unfavourable “one-star” reviews unless they received gift certificates. The same threats were made to eateries in Chicago and San Francisco and it appears that a vegan restaurant received as many as eight one-star reviews in the space of a week before being approached for money.
It’s surprising this sort of thing hasn’t emerged before. An over-reliance on the “wisdom of the crowd”, whereby many people measure things by the approval of the rest of the community, leaves us vulnerable to this kind of fraud.
It’s all about numbers. Products and companies are measured online by the number of stars they get on a five-star scale, influencers are measured by numbers of followers, posts are measured by the numbers of likes or retweets. The satirical Kardashian index provides a quantitative measure for academics by comparing citations of their research papers with their number of Twitter followers.
But why are these systems considered to be of value and why do we consult them almost blindly? In an age of information overload, feedback and reputation systems enable fast decision-making, providing us with the sense (or illusion) that we are in control as the decision taken is perceived to be informed.
Another idea at play here is the “attention economy paradigm”. Under this way of thinking, human attention is a scarce commodity and – as with all resources that are limited on this planet – it is of high value.
Businesses compete for a high as possible place on the first page of Google’s search results in order to capture this attention. And user feedback is one of the many parameters that influence the search engine’s secret ranking algorithms.
The notable success and acceptance of such reputation systems is grounded in the idea of the wisdom of the crowd comes in. If a sufficiently large sample of the population is asked to estimate something, the average of these estimations is expected to be very close to the actual value. This is because any personal bias becomes insignificant when a considerable amount of opinions is collected.
But all systems that come along with successful business models are open to abuse and can attract opportunistic and malicious actors, to an extent that organised criminal groups may form and systematically exploit such systems. For example, business opportunities that emerged during the COVID-19 pandemic were instantly matched by an assortment of criminal activities including shopping scams, disinformation, illegal streaming and even child sexual exploitation.
Fake reviews
There are several reasons and motivations for fake reviews. Business competitors may try to flood a business target with negative reviews in order to harm their competitor. Others may attempt, by creating fake profiles or “bribing” customers with free or discounted products, to engineer positive reviews and misrepresent the quality of their products.
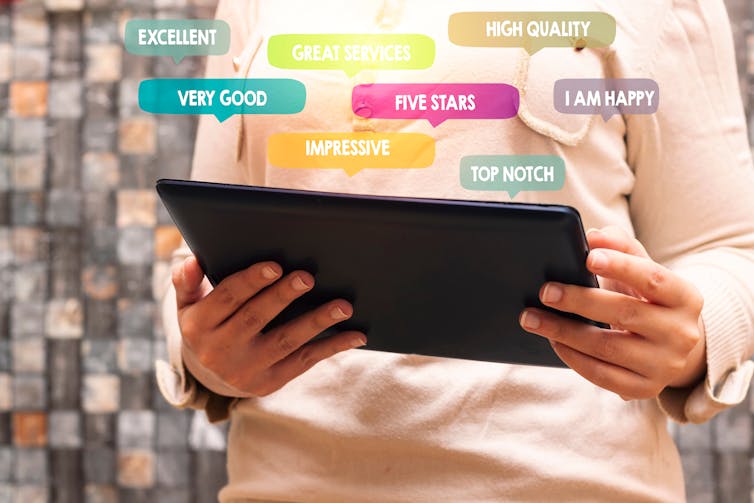
kheira benkada via Shutterstock
But extortion via threats of negative review is particularly insidious. A surge of negative reviews on a business’s Google profile not only affects its search engine ranking, but significantly influences the potential customers’ purchase decisions.
Although these practices are reported to have been streamlined from organised groups in India, variations of this have also been observed from other countries. Amazon recently sued 10,000 Facebook group administrators exceeding 43,000 members who allegedly solicit fake (positive) reviews in exchange for free products.
What can be done?
The abuse of online feedback and reputation systems has grown to epidemic proportion. Countering it will require the coordination of everyone involved.
Google and other feedback and reputation service providers need to invest more resources into the prevention, detection and removal of fake reviews. Machine learning technologies have made impressive leaps in recent years and could help in weeding out fake content.
Tighter rules governing the selection of reviewers enabling their participation under specific conditions. We’ve seen this with verified buyer schemes that aim to provide assurances that the reviewer has had a genuine experience with the business.
The presentation of the feedback and particularly the star scoring system could also have more contextual information, say through additional colour coding to communicate the sentiment mined out of the textual comments. In this case, highly emotional comments based on less factual or useful information could have a different colour from those trying to be impartial and objective.
Businesses also need to embrace the system for reporting problem reviews and use it responsibly. They should not report negative feedback if it is genuine, as this affects the relationship with the feedback platform, which will understandably be more distrustful to the business.
And consumers should be more alert and educated about this rather than following these rankings religiously. There are many telltale signs of a fake review, including simply checking the language to see if they are generic. It’s also instructive to check whether the reviewer produces a lot of negative reviews across many and seemingly unconnected products in a short time.
We, the crowd should be active participants by being always fair with our purchase experiences and acknowledge and support business when they exceed our expectations – but also provide candid negative reviews and recommendations for improvement. Only then the wisdom of the crowd will truly serve us.
Vasilis Katos, Professor of Cybersecurity, Head of BU-CERT, Bournemouth University
This article is republished from The Conversation under a Creative Commons license. Read the original article.